Blogs / Deep Learning vs Generative Models: An AI Face Off
Deep Learning vs Generative Models: An AI Face Off
Admin / February 22, 2024
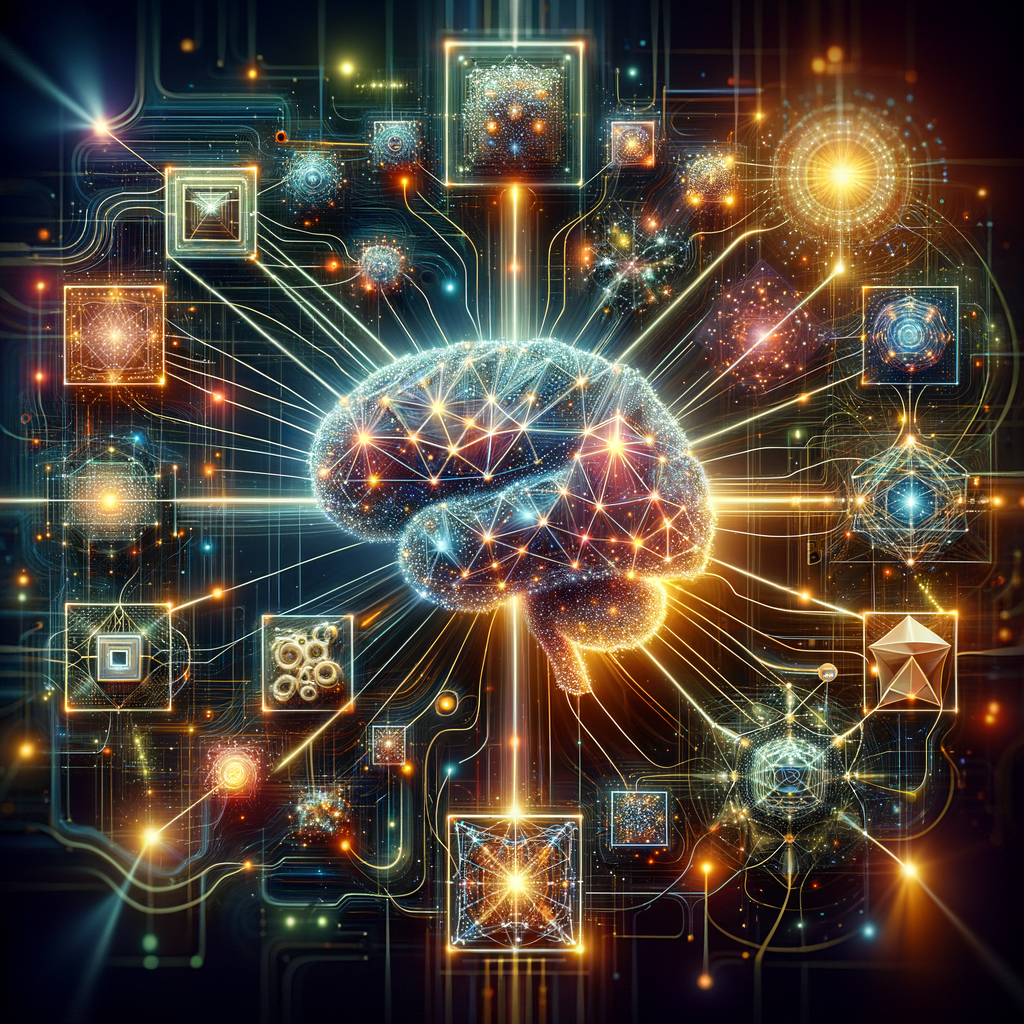
Exploring the Concepts: Deep Learning and Generative Models
As we delve into the comparative analysis of deep learning and generative AI techniques, it is essential to understand the basics of these two powerful methodologies. Deep learning, a subset of machine learning, utilizes neural networks with several layers (hence the 'deep' in deep learning) to model and understand complex patterns in datasets. This technique has been instrumental in achieving breakthroughs in areas like image recognition, natural language processing, and speech recognition.On the other hand, generative models, also a part of the machine learning framework, are designed to generate new data instances that resemble the training data. These models have found their niche in tasks like creating realistic images, enhancing image resolution, and generating human-like text.
In the AI face-off between deep learning and generative models, both these techniques bring unique strengths to the table. Deep learning excels in tasks that involve large datasets and require the extraction of intricate patterns. On the other hand, generative models shine in tasks that require the generation of new data instances based on learned data distributions.
Machine Learning in AI: An Analysis
In the realm of artificial intelligence, both deep learning and generative models have carved out their distinct roles. A comparative analysis of these AI techniques reveals their diverse applications and strengths.Deep learning techniques, with their ability to learn from large amounts of data, have been pivotal in developing highly accurate predictive models. These models have found applications in various fields, from healthcare diagnostics to autonomous driving. The power of deep learning lies in its ability to automatically learn data representations, eliminating the need for manual feature extraction.
Generative models, on the other hand, are known for their ability to generate new data instances that can pass as real data. This unique capability has led to the development of innovative applications, such as deepfakes, super-resolution images, and even AI-generated art.
In the AI face-off, the evaluation of deep learning techniques often revolves around their predictive accuracy and ability to handle large datasets. Generative models, however, are evaluated based on their ability to generate realistic data instances.
Comparing AI Techniques: Deep Learning and Generative Models
In the comparative analysis between deep learning and generative AI, it's clear that these techniques serve different purposes and excel in different areas. Deep learning algorithms are typically used for supervised learning tasks, where the aim is to learn a mapping from inputs to outputs. These techniques have been successful in tasks that involve complex pattern recognition, such as image classification and speech recognition.Generative models, on the other hand, are typically used for unsupervised learning tasks, where the goal is to learn the underlying data distribution. These models have been successful in tasks that involve generating new data instances, such as creating realistic images or synthesizing human-like text.
The AI face-off between deep learning and generative models is not about which technique is superior, but rather about understanding their unique strengths and applications.
Evaluating the Efficacy of Deep Learning and Generative Models
When it comes to the comparative analysis of deep learning and generative AI techniques, evaluating their efficacy is crucial. Deep learning techniques are often evaluated based on their predictive accuracy, generalization ability, and computational efficiency. These techniques are known for their ability to handle large datasets and extract intricate patterns, making them ideal for tasks that require high predictive accuracy.Generative models, on the other hand, are evaluated based on their ability to generate realistic data instances. These models are known for their ability to learn the underlying data distribution and generate new data instances that resemble the training data. This makes them ideal for tasks that require the generation of new data instances, such as image synthesis or text generation.
In the AI face-off, deep learning and generative models serve different purposes and excel in different areas. Therefore, the evaluation of these techniques should be based on their respective applications and strengths.
The AI Face Off: Which Holds the Future, Deep Learning or Generative Models?
In the comparative analysis of deep learning evaluation and generative AI techniques, the question arises: which holds the future? Both these techniques have shown immense potential and have revolutionized various fields.Deep learning, with its ability to handle large datasets and extract intricate patterns, has been instrumental in developing highly accurate predictive models. These models have found applications in various fields, from healthcare diagnostics to autonomous driving.
Generative models, with their unique ability to generate new data instances, have led to the development of innovative applications, such as deepfakes, super-resolution images, and AI-generated art.
In the AI face-off between deep learning and generative models, it's clear that both these techniques hold immense potential for the future. The choice between these techniques depends on the specific task at hand and the requirements of the application. In the world of AI, deep learning and generative models are not competitors, but rather complementary techniques that together will shape the future of artificial intelligence.